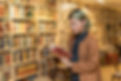
What the Research Says
Bridging historical insights with contemporary AI challenges
​
While freely accessible research specifically addressing generative AI in education remains limited, there exists a wealth of relevant insights from previous decades that could inform our present challenges. Join Professor Rose Luckin as she explores what the research says.
What the Research Says
"What the Research Says" - Bridging Historical Insights with Contemporary AI Challenges
​
A significant challenge has emerged in the conversations surrounding AI in education: despite decades of valuable research, there remains a notable scarcity of readily accessible, robust evidence regarding AI's current educational impact. This gap becomes particularly pronounced as we navigate the integration of generative AI into educational settings.
​
There is a disconnect between contemporary discussions and the rich historical understanding that has emerged over many decades of AI in education research. While freely accessible research specifically addressing generative AI in education remains limited, there exists a wealth of relevant insights from previous decades that could inform our present challenges.
​
Join Professor Rose Luckin as she explores what the research says...
What the Research Says: April 2025 - What the research says about: Self-Directed and Self-Regulated Learning in an AI-Driven World
​
Introduction​
​
In today's rapidly evolving educational landscape, two interconnected concepts have emerged as essential for effective learning: self-directed learning (SDL) and self-regulated learning (SRL). These approaches to learning are not merely academic constructs but vital skills for navigating a world where knowledge is abundant, constantly changing, and increasingly mediated by artificial intelligence.
​
Self-directed learning refers to the process where individuals take initiative and responsibility for their own learning journey. This encompasses identifying learning needs, establishing goals, locating appropriate resources, managing time effectively, and evaluating progress. As research spanning several decades has shown, SDL represents more than just independent study—it embodies a proactive mindset toward personal development that extends well beyond formal education settings.
​
Self-regulated learning, while closely related, focuses more specifically on the cognitive, metacognitive, and motivational processes learners employ within specific learning activities. SRL involves planning how to approach tasks, monitoring comprehension, evaluating progress, and adapting strategies when necessary.
​
Central to self-directed learning is the concept of self-regulation, which encompasses cognitive, metacognitive, and motivational dimensions. Self-regulated learning consists of:
​
-
Cognitive elements: The intentional implementation of learning processes and strategies
-
Metacognitive elements: Components that require learners to consider the process of learning, such as task analysis, goal setting, strategy selection, and monitoring
-
Motivational elements: Factors that impact and are affected by self-regulated learning, such as persistence and confidence
These components interact cyclically through phases of forethought, performance/volitional control, and self-reflection (Zimmerman, 2000), forming a comprehensive framework for understanding how learners regulate their learning processes. The importance of social contexts in learning regulation is also recognised, identifying three levels:
​
-
Self-regulation: Individual management of learning processes
-
Co-regulation: One person supporting another's self-regulatory processes
-
Socially shared regulation: Collaborative regulation where individuals coordinate regulation processes
This social dimension is particularly relevant for technology-enabled learning environments.
​
The significance of these sophisticated, thinking and learning capabilities has grown in recent years. In a world where information is ubiquitous and career trajectories rarely follow linear paths, the ability to direct and regulate one's learning has shifted from advantageous to essential. Traditional educational models, designed for knowledge scarcity and predictable career paths, struggle to prepare learners for environments characterized by information abundance and rapid technological change.
​
The emergence of artificial intelligence only accelerates this trend. As AI systems increasingly automate routine cognitive tasks, the premium on higher-order thinking skills and adaptability grows. The future belongs not to those who possess static knowledge but to those who can continuously identify what they need to learn, efficiently acquire new skills, and thoughtfully integrate new information with existing understanding.
​
As we design learning environments, create educational technologies, and shape educational policy for an AI-infused future, we face a fundamental question: Will our approaches empower learners to become more self-directed and self-regulated, or will they foster dependency and diminish these essential capabilities?
​
The answers to these questions will shape not just educational outcomes but the very nature of human agency and potential in a technology-mediated world. By understanding the mechanisms of SDL and SRL, we can develop approaches that harness technology—including AI—to enhance rather than replace human learning capabilities, creating a future where technology amplifies rather than diminishes human potential.
​
Key Researchers and Contributions
​
Research into SDL and SRL has evolved significantly over recent decades:
-
Barry Zimmerman developed a cyclical model of SRL, highlighting phases of forethought, performance, and self-reflection.
-
Paul Pintrich integrated cognitive, metacognitive, and motivational strategies within SRL frameworks.
-
Winne and Hadwin introduced a detailed model focusing on conditions, operations, products, evaluations, and standards (COPES), showing how learners interact with dynamic contexts.
-
Monique Boekaerts emphasised the importance of personal goals, emotional regulation, and socio-cognitive aspects of learning.
-
Roger Azevedo and colleagues advanced research on technology-supported SRL, using real-time data and adaptive feedback.
-
Hadwin, Järvelä, and Miller led research into social aspects of learning regulation, including co-regulation and socially shared regulation of learning.
​
Technology and SDL
​
Technology has transformed how self-directed learning can be implemented and supported. The integration of technology into SDL environments has evolved from simple computer-based learning modules to sophisticated AI-powered systems that can adapt to individual learner needs and behaviours.
​
Early applications of technology in SDL focused primarily on providing access to learning materials and resources. However, as technology has advanced, more interactive and adaptive systems have emerged, offering personalized learning experiences and real-time feedback.
​
Current Technological Approaches
​
Recent research reveals several technological approaches to supporting SDL:
​
-
Adaptive Learning Systems: These technologies use algorithms to adjust content difficulty and presentation based on learner performance (Voss et al., 2011)
-
Learning Analytics Platforms: These tools collect and analyse data on learner behaviours to provide insights into study patterns and effectiveness (Winne & Hadwin, 2013)
-
Virtual and Augmented Reality: Immersive technologies that allow learners to explore complex concepts in three-dimensional, interactive environments
-
Mobile Learning Applications: Apps designed to facilitate on-the-go learning, providing flexibility in when and where learning occurs
-
Intelligent Tutoring Systems: Computer-based systems that mimic human tutors, offering personalized instruction and feedback
-
Web 2.0 Tools: As highlighted by Sumuer (2018), these collaborative tools play a significant role in supporting self-directed learning with technology, enabling greater learner autonomy and engagement
​
Artificial Intelligence in SDL
Artificial intelligence has emerged as a particularly powerful tool for enhancing self-directed learning. Several AI approaches have been developed to support various aspects of SDL:
​
-
Pedagogical Agents: These virtual characters interact with learners, providing guidance and support during the learning process. As noted by Graesser and McNamara (2010), pedagogical agents can "more consistently promote SRL versus human tutors" (p. 67) by accurately tracking dialogue moves and computing optimal next steps.
-
Active Learning Algorithms: Inspired by machine learning, these algorithms select training examples that maximize learning efficiency. As Castro et al. (2008, cited in Gureckis & Markant, 2012) demonstrated, such approaches can significantly reduce the amount of training needed to achieve proficiency.
-
Recommendation Systems: AI-powered systems that suggest relevant resources based on learner preferences, past behaviour, and learning goals.
-
Natural Language Processing: Technologies that understand and respond to learner questions and comments, facilitating more natural interactions with learning systems.
​
Benefits of AI for SDL
​
Research has identified several advantages of using AI to support self-directed learning:
​
-
Personalisation: AI can analyse individual learning patterns and preferences to provide tailored content and feedback. This addresses what Atkinson (1972) identified as a key challenge in education: the need to optimize learning sequences for individual learners.
-
Scaffolding and Fading: AI systems can provide guidance when needed and gradually withdraw support as learners develop competence, supporting the development of self-regulatory skills.
-
Real-time Feedback: AI can offer immediate responses to learner actions, helping them understand their progress and make adjustments to their learning strategies.
-
Metacognitive Support: Advanced AI systems can prompt reflective thinking and self-assessment, fostering metacognitive development that is crucial for effective SDL.
​
A key advantage of AI in supporting SDL relates to how it can help learners gather information effectively. Gureckis and Markant (2012) highlight that AI algorithms can help learners optimise their information sampling by:
​
-
Uncertainty Reduction: Guiding learners toward information that reduces their uncertainty about a topic
-
Intervention-Based Learning: Supporting causal learning through experimentation and hypothesis testing
-
Exploration-Exploitation Balance: Helping learners navigate the trade-off between exploiting known information and exploring new areas
​
Effectiveness and Outcomes
​
Studies examining the effectiveness of technology-enhanced self-directed learning have generally shown positive results, though the findings are not uniform:
​
-
Knowledge Acquisition: Several studies have demonstrated improved content knowledge when learners use technology to support self-directed learning (e.g. Voss et al., 2011)
-
Retention and Transfer: Research by Markant and Gureckis (2010) found that self-directed learning supported by technology can lead to better retention and transfer of knowledge to new contexts
-
Self-Regulatory Skills: Technology-supported SDL has been shown to enhance metacognitive abilities and self-regulation strategies (Winne & Hadwin, 2013)
-
Learner Engagement: Multiple studies report increased motivation and engagement when learners have control over their learning process through technology tools
​
The effectiveness of technology and AI in supporting SDL appears to be mediated by several factors:
​
-
Learner Characteristics: Individual differences in prior knowledge, metacognitive abilities, and learning preferences impact how effectively learners can utilize technology for SDL
-
Technology Design: The usability, accessibility, and adaptability of the technology significantly influence its effectiveness in supporting SDL
-
Implementation Context: The social, cultural, and institutional context in which the technology is implemented shapes how it is used and its impact on learning
-
Task Complexity: The nature and complexity of the learning task affect how helpful technology support can be for self-directed learners
-
Self-Efficacy: As demonstrated by Sumuer (2018), both computer self-efficacy and online communication self-efficacy play significant roles in determining how effectively students can engage in self-directed learning with technology
​
The Impact of Generative AI on SDL
​
The rapid evolution of Generative Artificial Intelligence (GenAI) has created unprecedented opportunities for transforming educational paradigms. However, it is early days and there is no solid body of evidence or longitudinal research studies from which we can learn. But there are an emerging set of research reports that can be informative. By synthesising findings from these recent studies, published between 2022 and 2025, we can start to identify key themes, controversies, and knowledge gaps in this dynamic field.
​
Key Themes
Theme 1: GenAI as a Potential Enhancement for SDL
​
GenAI tools show potential to enhance SDL processes. Traditional SDL activities such as seeking resources, setting learning goals, and designing learning plans can be assisted by tools like ChatGPT (Li et al., 2024, cited in Roe & Perkins, 2024). Studies have demonstrated positive relationships between GenAI use and improved learning motivation in SDL contexts.
​
Wang et al. (2024) found that postsecondary learners primarily use ChatGPT for brainstorming and seeking inspiration for writing. Their study noted that ChatGPT alleviates social pressure by providing instant feedback, encouraging more writing practice and revision. Li et al. (2024) revealed that language learners develop adaptive strategies with ChatGPT, continuously adjusting their interactions to optimize learning outcomes.
​
Theme 2: The Educator as a GenAI Guide
​
The literature emphasizes educators' continued importance in AI-enhanced SDL environments. Wu et al. (2024) describe a bidirectional relationship, categorizing it into "AI-supported Teacher" and "Teacher-supported AI" approaches. In GenAI-enhanced environments, teachers must "relinquish authority and take a facilitative role" while developing skills to guide students in establishing learning goals and utilizing AI tools effectively.
​
Educators should adopt facilitative roles in AI-enhanced learning environments, guiding learners in optimizing their interactions with GenAI while fostering SDL skills. There is a need to cultivate learners' AI literacy, digital skills, and strategic approaches to integrating AI into learning processes.
​
Theme 3: Personalisation of Learning
GenAI's capacity to deliver personalised learning experiences emerges as a significant advantage for SDL. Multiple studies highlight that GenAI can generate adaptive content tailored to individual learning needs and preferences. Wu et al. (2024) found that technology acceptance significantly impacts self-directed learning ability, and personalized learning experiences allow highly motivated students to more effectively harness resources for autonomous learning.
​
Wang et al. (2024) describe how learners develop strategies such as designing specific prompts, pre-prompting with context, and fine-tuning questioning techniques to better direct their learning with AI. Learners who view AI as a useful tool demonstrate higher levels of engagement with these technologies.
​
Theme 4: Approaching with Caution
​
Researchers consistently emphasise the need for cautious implementation of GenAI in SDL contexts. Challenges include preventing over-reliance on technology and addressing the hallucination effect, where AI generates incorrect information. Wu et al. (2024) acknowledge that "overreliance on technology without critical thinking can detrimentally affect students' learning," and emphasize developing critical AI literacy.
​
Wang et al. (2024) found that while survey respondents demonstrated relatively low self-monitoring in AI-assisted learning, interviewees emphasized the importance of critically validating AI-provided information. Li et al. (2024) identify challenges including the "learning optimisation gap" and the "knowledge comprehension gap" between AI-generated content and learners' capacity to integrate it into their existing knowledge framework.
​
Challenges and Limitations of AI and Technology in SDL
Technological Challenges
​
Despite the potential benefits, several technological challenges limit the effectiveness of AI and technology in supporting self-directed learning (SDL):
​
-
Algorithm Limitations: As Gureckis and Markant (2012) note, "if the learning model is incorrectly specified for the domain... the information samples acquired will be severely biased" (p. 471). This can lead to ineffective or even harmful learning experiences.
-
Data Privacy Concerns: Collection of learner data raises significant privacy issues that must be addressed.
-
Digital Divide: Unequal access to technology creates disparities in who can benefit from technology-enhanced SDL.
-
Technical Reliability: Issues with software, hardware, or connectivity can disrupt the learning process.
​
Pedagogical Concerns
Several pedagogical challenges also emerge when implementing technology and AI for SDL:
​
-
Over-reliance on Technology: Learners may become overly dependent on technological scaffolding, potentially reducing their active intellectual engagement in the learning process and limiting the development of autonomous learning skills.
-
Superficial Engagement: Technology-enhanced environments may encourage surface-level interaction rather than deep learning.
-
Confirmation Bias: As highlighted by Gureckis and Markant (2012), self-directed learners may seek information that confirms existing beliefs rather than challenging them.
-
Cognitive Overload: Complex technological environments may overwhelm learners' cognitive resources, particularly for novices in a domain.
-
Lack of Training: Students need comprehensive support to effectively engage in self-directed learning with technology, specifically :
-
Self-directed learning skills: Helping students develop the ability to identify learning needs, set goals, select appropriate strategies, and evaluate outcomes.
-
Technical competencies: Building students' proficiency with the tools and platforms used for learning.
-
Self-efficacy development: Fostering students' confidence in their ability to use technology for learning and to communicate effectively in online environments.
-
​
Controversies and Tensions
AI Implementation vs. Educational Value
​
A significant controversy surrounds whether Generative AI (GenAI) truly enhances educational outcomes or merely changes the learning process without substantive improvements. Chun et al. (2025) found no statistically significant differences in academic achievement between traditional and AI-enhanced learning groups.
​
The tension between learning and assistance is notable, with concerns that learners may become overly reliant on AI tools, potentially reducing their active intellectual engagement in the learning process.
​
Human-AI Balance in Learning Environments
​
Another controversy concerns the optimal balance between human instruction and AI assistance. While educators remain central to the learning process, their role is transforming significantly. This creates tension around preserving valuable human elements of education while leveraging AI capabilities.
​
Equity and Access Concerns
​
Significant tensions exist regarding equitable access to AI-enhanced learning opportunities. Studies have not adequately addressed "the inbuilt biases, cultural orientation, and equity concerns that are part of AI in education" (Roe & Perkins, 2024). This raises questions about whether GenAI might exacerbate existing educational disparities.
​
Significant Gaps in Current Knowledge
​
One notable gap is the disproportionate focus on higher education applications of GenAI for SDL, with relatively few studies investigating K-12 settings. K-12 students face unique challenges in SDL compared to adults: "effective self-directed learning for younger students has two major influences: internal 'self' influences and external 'other' influences." Younger learners "need more guidance on what, why, where, and how of learning" (Ali et al., 2023).
​
Most current studies provide snapshots of implementation effects rather than tracking developmental trajectories over extended periods. Roe and Perkins (2024) explicitly call for "longitudinal studies to assess the long-term impact of the GenAI on SDL outcomes across multiple educational and cultural contexts."
​
The literature predominantly focuses on text-based GenAI applications, neglecting the broader possibilities of multimodal GenAI.
​
While multiple studies theorize about GenAI's potential benefits for SDL, robust empirical evidence demonstrating improved learning outcomes remains limited. More rigorous experimental studies are needed to establish causal relationships between GenAI use and improved learning outcomes.
​
The current literature lacks sufficient exploration of how GenAI-enhanced SDL functions across diverse cultural contexts and learner populations.
​
Guidelines for Educators
​
Educators seeking to support SDL through technology and AI should:
​
-
Balance facilitation with guidance: Relinquish some authority while still providing essential guidance. Help students establish clear learning goals and develop strategies for utilizing AI tools effectively.
-
Design AI-enhanced SDL environments: Create structured learning spaces that promote autonomy while incorporating AI tools, allowing learners to set goals, plan learning paths, and reflect on progress with technological support.
-
Foster AI literacy and critical thinking: Explicitly teach students to critically evaluate AI-generated content, recognize potential biases or inaccuracies, and develop validation strategies that compare AI outputs with established sources.
-
Differentiate implementation by educational level: Recognize that K-12 students require more structured guidance in SDL compared to higher education learners. Younger students need additional support on the "what, why, where, and how of learning."
-
Integrate metacognitive scaffolding: Incorporate prompts that encourage learners to think about their strategies and learning processes, especially when interacting with AI tools.
-
Employ learning analytics thoughtfully: Use data from AI-enhanced learning to help learners visualize their progress and identify areas for improvement, while being transparent about how this data is collected and used.
-
Promote collaborative AI use: Encourage co-regulation and socially shared regulation of learning by designing collaborative activities where students can collectively interact with and learn from AI tools.
-
Adapt to evolving roles: Develop skills in "AI-supported teaching" and "teacher-supported AI" approaches, focusing on how to enhance both teaching effectiveness and student learning experiences.
-
Address equity concerns proactively: Recognize and mitigate potential barriers to equitable access, providing additional support for students who may have limited access to required technology.
-
Evaluate long-term impact: Regularly assess how AI implementation affects learning outcomes over time, rather than focusing solely on immediate results.
​
Guidelines for Technology Developers
​
Developers creating technology and AI tools to support SDL should:
​
-
Design for comprehensive SDL cycles: Support the complete self-directed learning process, including planning, monitoring, control, and reflection phases, rather than focusing on isolated components.
-
Enhance personalisation capabilities: Develop systems that deliver truly adaptive content tailored to individual learning needs, preferences, and contexts, allowing for customized learning pathways.
-
Bridge learning optimization gaps: Create interfaces and features that help learners fully leverage the technical affordances of GenAI, addressing the gap between AI capabilities and users' ability to utilize them effectively.
-
Incorporate evidence-based prompts: Include metacognitive scaffolds and prompts that guide learners through the SDL process, with adaptive fading as learners develop greater self-regulation capabilities.
-
Create transparent learning analytics: Develop analytics that help learners understand their own processes and progress while making the limitations of AI-generated insights clear.
-
Support multimodal learning: Extend beyond text-based interactions to incorporate visual, audio, and interactive elements that enhance understanding across different learning domains.
-
Balance automation with learner agency: Ensure AI provides suggestions and support rather than prescriptive pathways, preserving learner decision-making and ownership of the learning process.
-
Address cultural and contextual diversity: Design tools that can adapt to diverse cultural contexts and learner populations, recognising that SDL approaches may vary across cultural traditions.
-
Prioritize ethical data use: Collect only necessary data and be transparent about its use, addressing concerns about algorithmic biases, data privacy, and ideological influences.
-
Enable collaborative learning: Include features that support co-regulation and socially shared regulation of learning, facilitating collaborative SDL experiences.
-
Develop K-12 specific implementations: Create tools specifically designed for younger learners that provide appropriate levels of guidance and structure while still fostering SDL capabilities.
​
Guidelines for Policy Makers and Educational Leaders
​
Policy makers and educational leaders should:
​
-
Recognise SDL as a core competency: Update curriculum frameworks to position SDL as an essential 21st-century skill, particularly in AI-enhanced educational environments.
-
Invest in comprehensive teacher development: Provide substantial professional development focused on technology-enhanced SDL, helping educators transition to facilitative roles in AI-enhanced learning environments.
-
Develop ethical frameworks: Create comprehensive guidelines addressing privacy, bias, learner autonomy, and responsible AI use in educational settings.
-
Prioritise equity and access: Ensure technologies supporting SDL are available to all learners, addressing both technical access and support needs across diverse populations.
-
Support longitudinal research: Fund studies examining long-term impacts of AI-enhanced SDL across various educational contexts, age groups, and cultural settings.
-
Balance innovation with evidence: Encourage educational innovation while requiring evidence of improved learning outcomes, not just technological implementation.
-
Address K-12 to higher education continuity: Develop policy frameworks that support SDL development across the full educational spectrum, recognising different needs at various educational levels.
-
Promote cross-sector collaboration: Facilitate partnerships between educators, technology developers, researchers, and policymakers to ensure alignment of SDL approaches.
-
Consider sustainability dimensions: Evaluate AI implementations through environmental, social, and economic sustainability lenses, as outlined in recent research.
-
Create accountability frameworks: Establish mechanisms to evaluate whether AI tools are enhancing rather than diminishing SDL capabilities and educational quality
​
Why Supporting SDL Matters
The ability to direct one's own learning is essential in today's fast-changing world. Careers increasingly require continuous learning, complex problem-solving, and adaptability. Technology, particularly AI, offers significant potential to support SDL, but its effectiveness depends on thoughtful, research-informed implementation.
The case for the importance of SDL in today's world:
Rapid knowledge evolution: In fields where knowledge changes quickly, the ability to direct one's own learning is essential for ongoing relevance.
Lifelong learning demands: Career trajectories increasingly require continuous learning beyond formal education.
Complex problem-solving: Modern challenges require the ability to identify learning needs and acquire new knowledge and skills.
Digital transformation: Technology is transforming how learning happens, making SDL capabilities more important than ever.
Learner empowerment: Developing SDL shifts education from passive consumption to active ownership of learning.
Evolving educational roles: While educators remain central to the learning process, their role is transforming significantly toward facilitation and guidance in AI-enhanced environments.
Critical approach necessities: Research consistently emphasizes the need for developing critical AI literacy, addressing concerns about overreliance, misinformation, and algorithmic biases.
Equity considerations: Studies highlight tensions regarding equitable access to AI-enhanced learning opportunities, requiring deliberate policy and implementation approaches.
By aligning educational practices, technology design, and policy with established research on SDL and SRL, we can create environments that empower learners to take ownership of their learning journeys, preparing them for lifelong learning and success in an evolving digital landscape.
References
​
Ali, F., Choy, D., Divaharan, S., Tay, H. Y., & Chen, W. (2023). Supporting self-directed learning and self-assessment using TeacherGAIA, a generative AI chatbot application: Learning approaches and prompt engineering. Learning: Research and Practice. https://doi.org/10.1080/23735082.2023.2258886
​
Alshahrani, A. (2023). The impact of ChatGPT on blended learning: Current trends and future research directions. International Journal of Data and Network Science, 7(6), 2029–2040. https://doi.org/10.5267/j.ijdns.2023.6.010
​
Azevedo, R., Johnson, A., Chauncey, A., & Graesser, A. (2015). Use of hypermedia to assess and convey self-regulated learning. In Handbook of self-regulation of learning and performance (pp. 1-1237). https://doi.org/10.4324/9780203839010.ch7
​
Boekaerts, M. (2002). Bringing about change in the classroom: Strengths and weaknesses of the self-regulated learning approach—EARLI Presidential Address, 2001. Learning and Instruction, 12(6), 589–604. https://doi.org/10.1016/S0959-4752(02)00010-5
​
Chun, J., Kim, J., Kim, H., Lee, G., Cho, S., Kim, C., Chung, Y., & Heo, S. (2025). A comparative analysis of on-device AI-driven, self-regulated learning and traditional pedagogy in university health sciences education. Applied Sciences, 15(4), 1815. https://doi.org/10.3390/app15041815
​
Hadwin, A., Järvelä, S., & Miller, M. (2018). Self-regulation, co-regulation, and shared regulation in collaborative learning environments. In D. H. Schunk & J. A. Greene (Eds.), Handbook of self-regulation of learning and performance (pp. 83–106). Routledge/Taylor & Francis Group. https://doi.org/10.4324/9781315697048-6
​
Hadwin, A. F., Järvelä, S., & Miller, M. (2011). Self-regulated, co-regulated, and socially shared regulation of learning. In B. J. Zimmerman & D. H. Schunk (Eds.), Handbook of self-regulation of learning and performance (pp. 65–84). Routledge/Taylor & Francis Group.
​
Knowles, M. S. (1975). Self-directed learning: A guide for learners and teachers. Association Press.
​
Li, B., Bonk, C. J., Wang, C., & Kou, X. (2024). Reconceptualizing self-directed learning in the era of generative AI: An exploratory analysis of language learning. IEEE Transactions on Learning Technologies. https://doi.org/10.1109/TLT.2024.3386098
​
Pintrich, P. R. (2000). The role of goal orientation in self-regulated learning. In M. Boekaerts, P. R. Pintrich, & M. Zeidner (Eds.), Handbook of self-regulation (pp. 451–502). Academic Press. https://doi.org/10.1016/B978-012109890-2/50043-3
​
Pintrich, P. R., Smith, D. A., Garcia, T., & McKeachie, W. J. (1993). Reliability and predictive validity of the Motivated Strategies for Learning Questionnaire (MSLQ). Educational and Psychological Measurement, 53(3), 801-813. https://doi.org/10.1177/0013164493053003024
​
Pintrich, P. R., & Zusho, A. (2002). The development of academic self-regulation: The role of cognitive and motivational factors. In A. Wigfield & J. S. Eccles (Eds.), Development of achievement motivation (pp. 249–284). Academic Press. https://doi.org/10.1016/B978-012750053-9/50012-7
​
Roe, J., & Perkins, M. (2024). Generative AI in self-directed learning: A scoping review [Preprint]. arXiv. https://doi.org/10.48550/arXiv
​
Wang, C., Li, Z., & Bonk, C. (2024). Understanding self-directed learning in AI-assisted writing: A mixed methods study of postsecondary learners. Computers and Education: Artificial Intelligence, 6, 100247. https://doi.org/10.1016/j.caeai.2024.100247
​
Winne, P. H., & Hadwin, A. F. (1998). Studying as self-regulated learning. In D. J. Hacker, J. Dunlosky, & A. C. Graesser (Eds.), Metacognition in educational theory and practice (pp. 277–304). Lawrence Erlbaum Associates Publishers.
​
Winne, P. H., & Hadwin, A. F. (2013). nStudy: Tracing and supporting self-regulated learning in the Internet. In R. Azevedo & V. Aleven (Eds.), International handbook of metacognition and learning technologies (pp. 293-308). Springer. https://doi.org/10.1007/978-1-4419-5546-3_20
​
Wu, D., Zhang, S., Ma, Z., Yue, X.-G., & Dong, R. K. (2024). Unlocking potential: Key factors shaping undergraduate self-directed learning in AI-enhanced educational environments. Systems, 12(9), 332. https://doi.org/10.3390/systems12090332
​
Zimmerman, B. J. (1986). Becoming a self-regulated learner: Which are the key subprocesses? Contemporary Educational Psychology, 11(4), 307-313. https://doi.org/10.1016/0361-476X(86)90027-5
Zimmerman, B. J. (2000). Attaining self-regulation: A social cognitive perspective. In M. Boekaerts, P. R. Pintrich, & M. Zeidner (Eds.), Handbook of self-regulation (pp. 13-39). Academic Press. https://doi.org/10.1016/B978-012109890-2/50031-7
What the Research Says: Mid-February 2025 - Bridging the Science-Practice Chasm to Enhance Robust Student Learning
​
The next research for analysis is by researchers in Pittsburgh: Kenneth Koedinger, Albert T. Corbett, and Charles Perfetti. Their research aims to bridge the gap between cognitive science research and educational practice. A framework is presented that provides a systematic approach to understanding how students learn by analysing three key components:
1. Knowledge Components (KCs) - Units of cognitive function or structure that can be inferred from student performance
2. Learning Events (LEs) - Processes through which students acquire knowledge
3. Instructional Events (IEs) - Activities designed to facilitate learning
​
Research discussed:
What the Research Says: Late January 2025 - The 2 Sigma Problem: the Search for Methods of Instruction as Effective as One-to-One Tutoring
​
Following the full-on UK Bett Show 2025, for this week's 'What the Research Says', Professor Rose Luckin explores the seminal paper by Benjamin Bloom.
​
Research discussed:
What the Research Says: January 2025 - Early Lessons from AI Tutoring that Matter Today
​
For our first 'What the Research Says', Professor Rose Luckin explores early lessons from AI tutoring that matter today. Rose covers this in the January 2025 issue of the Skinny and is holding a live talk on the material at the UK Bett Show in London on 24th January. Please note this talk will not be recorded but future sessions will be held online.
​
Research discussed: